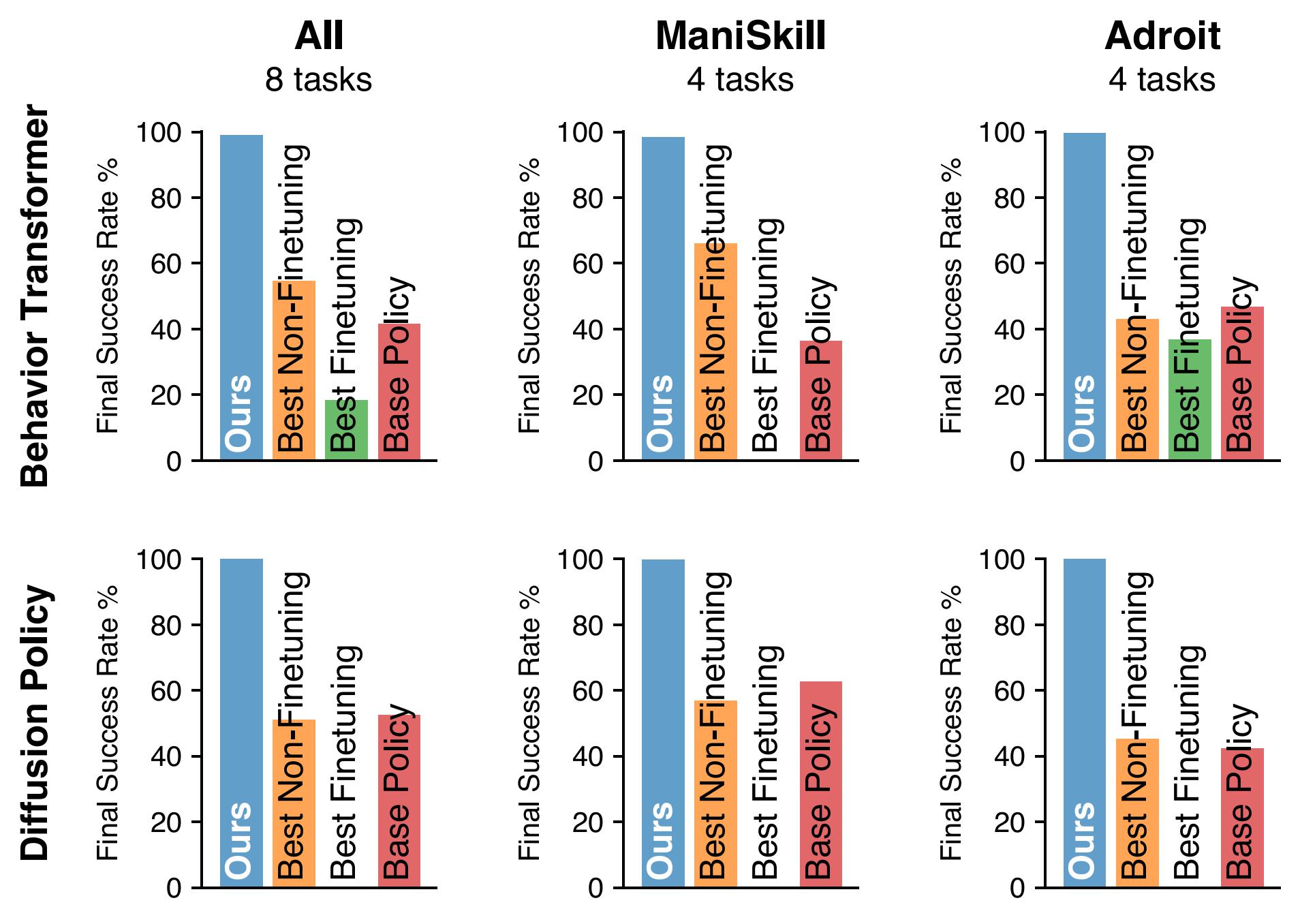
Overview. We propose Policy Decorator, a framework designed to enhance large policy models (e.g., Diffusion Policy and Behavior Transformer) through online environment interactions. Policy Decorator utilizes residual policy learning and controlled exploration strategies to achieve model-agnostic and sample-efficient performance improvements. As shown in the animation, while offline-learned policy struggles with the finer parts of the task—such as precisely inserting a peg into a hole—our refined policy achieves flawless insertions.
Click the "cc" button at the lower right corner to show captions.
The offline-trained base policies can reproduce the natural and smooth motions recorded in demonstrations but may have suboptimal performance.
Policy Decorator (ours) not only achieves remarkably high success rates but also preserves the favorable attributes of the base policy.
Policies solely learned by RL, though achieving good success rates, often exhibit jerky actions, rendering them unsuitable for real-world applications.
click blue texts for visualizations
What are the pros of Policy Decorator compared to base policy?
What are the pros pf Policy Decorator compared to RL policy?
JSRL policy also performs well on some tasks, why not use it?
What happens if we fine-tune base policy with a randomly initialized critic?